Tuesday May 9, 2023
10th Annual NJBDA Symposium
Big Data in FinTech
Host: Seton Hall University
The New Jersey Big Data Alliance (NJBDA) is an alliance of 18 higher education institutions, as well as industry and government members, that catalyzes collaboration in advanced computing and data analytics research, education and technology.
The NJBDA’s annual symposium is New Jersey’s premier conference for big data & advanced computing, consistently attracting 200+attendees from industry, government, and academia.
This year’s symposium will be held in person at Seton Hall University. Read Seton Hall’s press release.
Seton Hall University is located at 400 South Orange Ave., South Orange, New Jersey (Google map: https://goo.gl/maps/qoTCgk1kV9GENnZL6). South Orange can be reached by car and NJ Transit train and bus.
The conference venue is the University Center (Building 12 on the campus map: https://www.shu.edu/visit/upload/Campus_Map.pdf). Parking will be available on campus as directed at the gate.
For questions, please contact Manfred Minimair, Ph.D., Seton Hall. For sponsorship inquiries, please contact Kaerielle Larsen, Seton Hall, at kaerielle.larsen@shu.edu.
Keynotes
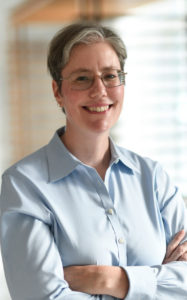
Kjersten Margaret Moody
Chief Data Officer, Prudential Financial
Get Ready to Create the Future
You’ve experienced the latest advancements in data and analytics. You’ve read the headlines about quantum computing, blockchain, generative AI, and more. But what comes next, and what do you need to do to prepare?
By attending this session led by Kjersten Moody, Prudential Financial’s Chief Data Officer, you’ll gain a deeper understanding of how your technical knowledge, leadership skills, and real-world experience can be the catalyst to prepare you for the transformation ahead in your careers and to succeed in driving business success and creating growth opportunities.
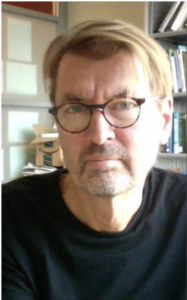
George Calhoun
Professor and Founding Director of the Quantitative Finance Program, Stevens Institute of Technology
New Jersey has all the elements to build a Fintech version of “Silicon Valley” – except a robust venture development eco-system. The State is currently ranked 10th in Fintech company formation. This could put incumbents at risk of significant disruption from new tech-enabled players from outside the traditional financial services industry (as has already happened in some segments, such as the exchange sector). The question is: What has to happen for the State to become a world center of Fintech innovation, entrepreneurship and job creation?
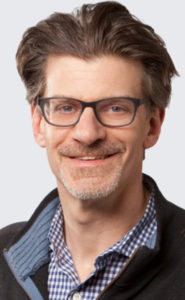
Marc Rind
Chief Technology Officer-Data, Fiserv
Agenda
8:30 – 9:30
Registration, Refreshments, Exhibits
Location: Event Lounge and Event Room
9:30 – 10:00
Welcome
- Matt Hale, President, NJBDA; Associate Professor, Seton Hall University
- Manfred Minimair, NJBDA Symposium Chair; Professor, Seton Hall University
- Anthony Loviscek, Professor, Seton Hall University, Academy of Applied Analytics and Technology
- Katia Passerini, Provost and Executive Vice President, Seton Hall University
Location: Event Room
10:00 – 10:45
Keynote 1: Kjersten Moody
Chief Data Officer of Prudential Financial
(30 minutes speech, 15 min Q&A)
Location: Event Room
10:45 – 11:00
Break, Exhibits (incl. Student Posters), Networking
The 2023 Symposium will include a student research poster session on the topic of Big Data in FinTech. Students from NJBDA member institutions and others are welcome to submit a poster. Topics related to the focus of the symposium theme include, but are not limited to, Large Language Models, AI, Finance, Blockchain, Crypto & Digital Currencies, NFTs, and Big Data Business Intelligence are also invited. Please submit your posters by April 14th, 2023, with a separate cover page, including your name, email, department, institutional affiliation, and title of the poster, to https://sites.google.com/stockton.edu/2023-njbda-symposium. Bring a 36” X48” printed copy of the poster to the event on May 9th, 2023.
Location: Event Lounge
- George Avirappattu, Associate Professor, Kean University (Organizer)
- Demetrios Roubos, Information Security Officer, Stockton University (Organizer)
11:00 – 12:00
Parallel Sessions:
AI/Machine Learning Applications
The need to adapt to newer technologies and cater to a wide customer base with customized needs has become the need of the hour, with companies constantly innovating. Successful companies have used AI-ML to design products suiting their customer’s evolving needs. Over the recent years, machine learning has had a major impact in the finance lending sector by allowing for more accurate and faster decision-making through analysis of consumer data, usage trends, and patterns.
This session will focus on the challenges and benefits of using such disruptive technologies of AI and ML for better BI on customers, more informed decision making, and improved risk management, and lower costs.
- Rashmi Jain, Professor, Montclair State University (Moderator)
- Bhupinder Bhullar, Managing Director and Co-Founder, Swiss Vault
- Jason Cooper, Chief Technology Officer, Paradigm
- Amitabh Patil, Co-Founder and CTO, Whiz AI
Location: Chancellor’s Suite
Data Assets and Privacy
The workshop considers data as an asset and examines implications for technological, legal and privacy frameworks. A case study from the Island of Jersey will be presented, generalizing the legal framework of trusts in Jersey to data assets. Data assets considered in the case study are personal activity data derived from cyclists on the island. The trusts serve as tools to preserve data ownership and privacy. The second in-depth study covers the use of data from electronic health care records. Such data is used for machine learning applications and statistical inference to support medical trials and to assess vulnerabilities of the data. It will be discussed how such applications can be compliant with privacy laws and will not compromise patient identity.
- David Opderbeck, Professor, Seton Hall University (Moderator)
- Tony Moretta, CEO, Digital Jersey
- Choudur Lakshminarayan, Teaching Professor, Stevens Institute of Technology
Location: Meeting Room 206
Entrepreneurship in FinTech
The fintech space is one of the fastest growing areas of entrepreneurship, with new startups popping up every day. In addition, big data has had a significant impact on the fintech industry revolutionizing the way financial services are delivered and changing the competitive landscape. Panelists will discuss the how they are using big data, the current market landscape, opportunities for disruption and the challenges that come from operating in a regulated environment. Given the recent turmoil in the banking industry, the panelists will also provide insights and perspectives on the current state of funding in fintech.
- Judith Sheft, Executive Director, New Jersey Commission on Science, Innovation and Technology (Moderator)
- Nancy Schneier, Chief Revenue Officer and Co-Founder, Vikar Technologies
- Yingchao Zhang, Head of Global Solutioning, AYR.ai
- Gabriel L Pauliuc, Chief Data and Technology Officer, HearRWorld, LLC
- Chisa Egbelu, Co-Founder and CEO, PeduL
Location: Event Room
12:00 – 1:00
Lunch, Exhibits (incl. Student Posters), Networking
Location: Event Lounge and Event Room
1:00 – 2:00
Parallel Sessions:
Workforce Development for FinTech
Talent and human capital are now, and will continue to be, the critical factors of production in the data-driven economy. Technology and lifelong training to maintain the appropriate skills become pre-conditions for participating in this new landscape. By educating, training, and facilitating access to individuals with advanced computing and analytics skill sets, New Jersey can provide a competitive advantage for its employers. This workshop will highlight the specific skills and workforce development needs of the fintech sector to ensure the successful growth of this critical sector for the state economy.
- William Noonan, Chief Business Development Officer, Choose New Jersey
- Mark Guthner, Professor of Financial Practice, Rutgers Business School
- Steven Hunter, Director, AI Center of Excellence and co-organizer of the UBS Pitch Competition, UBS
- Peggy Brennan-Tonetta, Director, Resource and Economic Development, New Jersey Agricultural Experiment Station, Rutgers, the State University of New Jersey (Moderator)
Location: Event Room
Cryptocurrencies and Risk Management
The panel will cover investigatory and criminal aspects of cryptocurrencies, risk management from the corporate perspective, and relevant underlying technologies such as blockchain. The discussion will explore how blockchain technology facilitates the identification of certain risks by providing positional information about counterparties, while also creating new risks due to the transparency of market participants’ information. AI-powered fraud detection systems will be discussed, with a focus on their ability to analyze large amounts of transaction data in real time to identify suspicious patterns or anomalies that may indicate fraudulent activity. By using machine learning algorithms, these systems can learn over time to identify increasingly sophisticated forms of fraud. Topics will include prevalent cyberattacks targeting financial institutions as well as the evolving risk landscape in the financial sector. The discussion will also delve into security risks and challenges associated with the ongoing digital transformation and the emergence of open and decentralized banking models.
- Demetrios Roubos, Information Security Officer, Stockton University (Moderator)
- Petter Kolm, Professor, NYU Courant
- Mert Saglam, Special Agent, US Secret Service
- Rodney Sunada-Wong, Risk Manager
Location: Chancellor’s Suite
Research Presentations in FinTech
The symposium Research Track features presentations on current applied research in Big Data, AI, and Machine Learning. Presentations are categorized based on specific symposium themes in Big Data and FinTech.
- Forough Ghahramani, Associate Vice President for Research, Innovation, and Sponsored Programs, Edge and NJBDA Vice President for Research and Collaboration Committee (Research Track Chair)
AI/Big Data Session
Session Moderator: Abhishek Tripathi, PhD, The College of New Jersey
Location: Meeting Room 206
FinTech Session
Session Moderator: Thanh Trung Nguyen, PhD, Rowan University
Location: Faculty Lounge
2:00 – 2:45
Keynote 2: George Calhoun
Director of the Quantitative Finance Program and the Hanlon Financial Systems Center at Stevens Institute of Technology
(30 minutes speech, 15 min Q&A)
Location: Event Room
2:45 – 3:15
Keynote 3: Marc Rind
Chief Technology Officer-Data, Fiserv
Location: Event Room
3:15 – 3:20
Journal of Big Data Theory and Practice
- Jim Samuel, Associate Professor of Practice, Executive Director – Informatics, Bloustein School of Planning and Public Policy, Rutgers University
Location: Event Room
3:20 – 3:30
Closing Remarks and Raffle
- Manfred Minimair, NJBDA Symposium Chair, Professor, Seton Hall University
- Matt Hale, President, NJBDA, Associate Professor, Seton Hall University
Location: Event Room
3:30 – 5:00
Post-Symposium Reception
Location: Chancellor’s Suite